What is the difference between Data Engineering and Data Science?
Data Engineering and Data Science are complimentary.
Data Engineering ensures that data scientists can look at data security and consistently. Data Engineers handle many core elements of Data Science, such as the initial collection of raw data and the process of cleansing, sorting, securing, storing, and moving that data.
Data Science combines computer science, statistics, and mathematics. Data Scientists apply a combination of algorithms, tools, and machine learning techniques like predictive analytic to help you to extract knowledge from the data.
What are the key Data Engineering technical skills?
Data Engineers need to know skills and tools like:
Python, Java, and Scala programming languages
Python is the top programming language used for Data Engineering, followed by Java which is widely used in data architecture frameworks (most of their APIs are designed for Java). Scala is an extension of the Java language that simplifies its syntax.
Database systems (SQL and NoSQL)
SQL is the standard programming language for building and managing relational database systems (tables made of rows and columns). NoSQL databases are non-tabular and come in a variety of types depending on their data model, such as a graph or document.
Data warehouses
Data warehouses store large volumes of current and historical data. This data is sourced from numerous sources, such as CRMs, ERPs, and accounting software. Data Engineering services help organizations extract knowledge from data through reporting, analytics solutions, and data mining.
Why are Data Engineers more than just experts on one specific technology?
The technologies used by Data Engineering companies use have quickly evolved over past two decades. Nowadays, data processing is more often done with technologies like Apache Spark, Apache Hive, Apache Kafka, and other big data technologies that are running on cloud platforms like Amazon Web Services or Google Cloud Platform.What are the non-technical skills that are the most valuable for Data Engineers?
Data Engineers need a handful of soft skills to perform their job well:
Communication skills
Data Engineering team will almost certainly interact with a diverse range of stakeholders, many of whom possess varying degrees of technical expertise. Communication skills are critical for effective collaboration.
Collaboration
As critical as communication abilities, Data Engineers must be able to work in teams. Data Engineers need to understand the expectations of Data Science teams with whom they are collaborating, the frequency with which they require updates, and their pain points.
Adaptability
As projects change or evolve, they must be able to reprioritize and adjust. When things do not go according to plan, Data Engineering experts must be able to devise a workaround. Failure to do so may result in frustration, missed deadlines, and resource wastage.
How do you typically deploy a Data Engineering solution?
At a high level, Big Data Engineering has a generic architecture that applies to the majority of businesses:
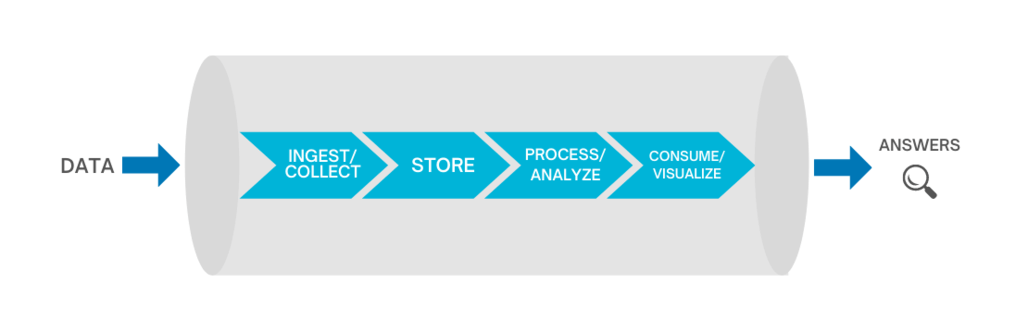
Data Ingestion
You need your Big Data setup to handle all incoming data streams, whether structured, unstructured, or semi-structured. The incoming data is prioritized and categorized for a smooth flow into further layers down the line. Data ingestion can happen through real-time streaming or batch jobs. We typically use Apache Kafka and AWS/GCP specific solutions (GCP Pub/Sub, GCP Big Query, GCP Cloud Storage, AWS Redshift, AWS S3, AWS Athena, etc) for creating data ingestion pipelines.
Data Storage
After raw data is ingested, the extracted data should be stored somewhere. The storage solution should be in line with the data ingestion requirement of your business ecosystem.
Data Processing
The processing layer where the analytical process begins, where data is needed for analysis is selected, cleaned, formatted for further analysis and modeling. The goal is to discover useful information, suggest conclusions,s and support decision-making.
Data Processing on AWS by access characteristics:
Data Visualizations
This layer is everything to do with a graphical representation of information and value gained through analysis. Using rich charts, graphs, and maps, the tools in this layer help present a compelling story for a decision to be made by your leadership team.
We typically use Amazon QuickSignt or Tablau, see our article Embedded Analytics: Amazon QuickSight vs Tableau